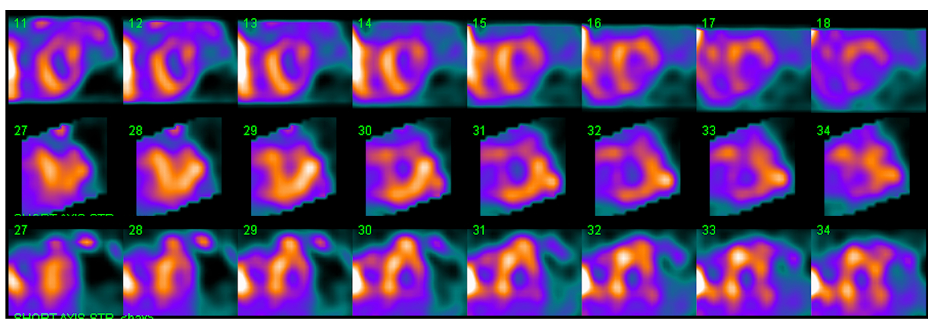
Cardiac Amyloidosis Discovery
Changing the natural history of cardiac amyloidosis through earlier detection
Cardiovascular disease remains the leading cause of death in the United States. Significant disparities exist in cardiovascular disease by race, ethnicity, and socioeconomic status, including in the prevalence of risk factors, the risk of specific cardiovascular conditions such as heart failure, and in access to diagnostic tools such as echocardiography. Failure to diagnose cardiovascular disease represents a significant burden of preventable cardiovascular disease and an opportunity to improve health and advance justice within the healthcare system.
Transthyretin cardiac amyloidosis (ATTR-CA) is an underrecognized disorder that disproportionately affects Hispanic and non-Hispanic Blacks who have both a higher prevalence of disease due to an underlying genetic variant and worse survival after diagnosis compared to other populations. Although it is marked by ECG and echocardiographic changes with decreasing ECG voltage and increasing LV wall thickness, ATTR-CA has historically been diagnosed late in the disease course due to nonspecific signs and symptoms of early-stage disease.
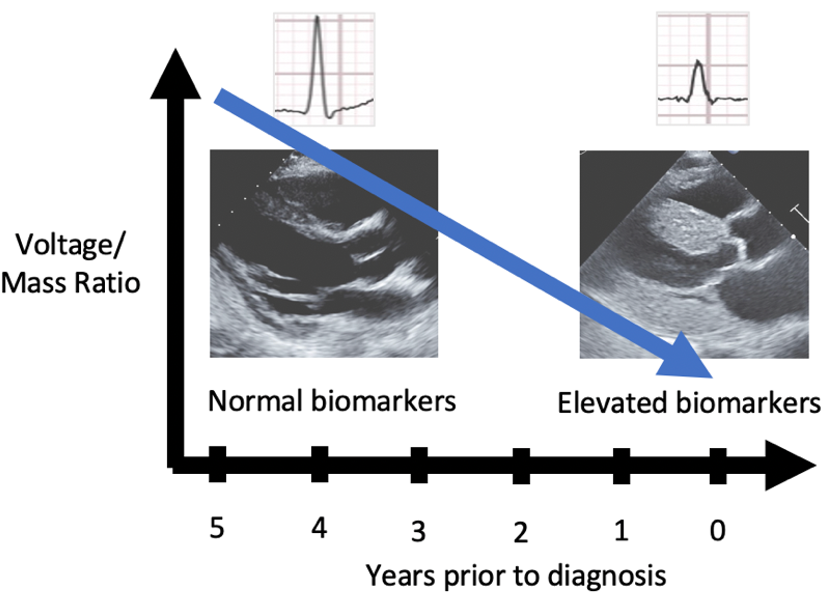
In an effort to improve the early detection of cardiac amyloidosis when it is most treatable, we have developed a deep learning model using the ECGs and echocardiograms of ~1,000 patients who have been tested for cardiac amyloidosis. The core model uses a convolutional neural network to analyze the ECG waveform with tabular LV measures from the echocardiogram fused into the model to yield a final model prediction.
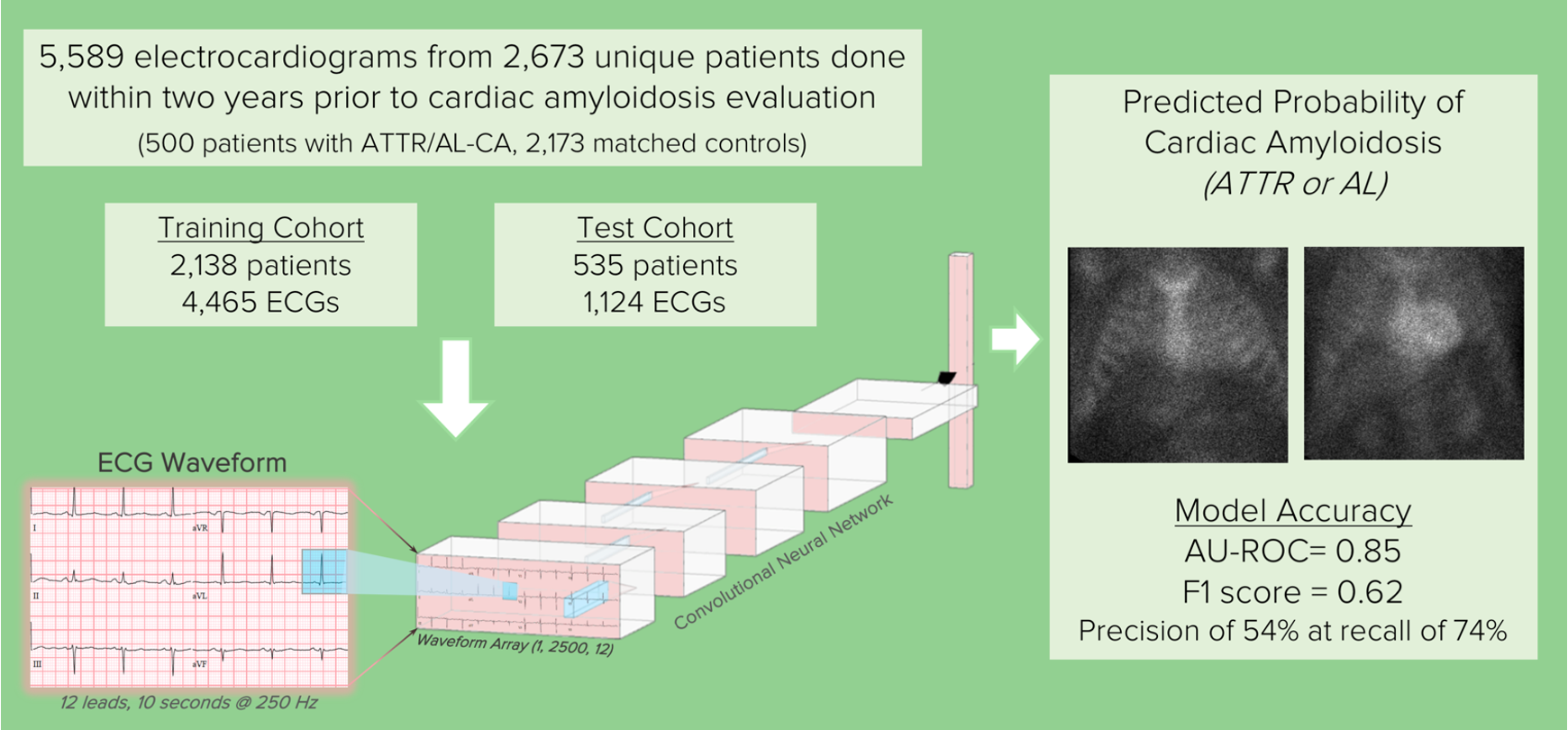
We are currently carrying out a 100-patient prospective clinical study using this technology. By applying the deep learning model to every patient at our medical center who has undergone an ECG and an echocardiogram, we can identify the patients at highest risk for having undiagnosed cardiac amyloidosis. These patients are then recruited for testing with the hope that this model will lead to earlier detection and improved outcomes.